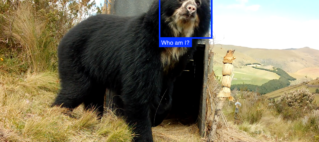
Bringing BearID to Ecuador
I spent a 4-week sabbatical from Arm with co-director, Mary Nguyen, helping researchers in Ecuador accelerate their camera trap analysis with AI. Our main collaborator
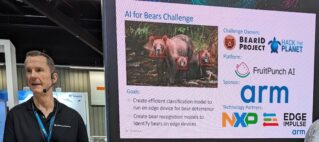
AI for Bears Challenge
In April of 2024, the BearID Project hosted an AI for Bears Challenge with FruitPunch AI. The challenge was sponsored by Arm and included partners
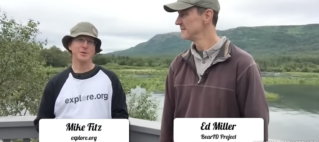
BearID Project update on Explore.org
In July of 2023, I was interviewed Mike Fitz, Resident Naturalist for Explore.org at Katmai National Park in Alaska. The interview was included in a